The AI Action Figure Fiasco: How OpenAI's Transparency Problem Makes Bias Even Worse
Oh look, another AI breakthrough that works perfectly... if you're white. And now, another AI release without the safety documentation we were promised. See a pattern? I sure do.
What's that? You're not white? Well, prepare to be transformed into whatever ethnically ambiguous stereotype the algorithm decided looks "close enough" to you! In my case, apparently that's "Black Female Jensen Huang" – a transformation so wildly off-base it would be laughable if it weren't so disturbing.
The Great Action Figure Divide
While social media, especially LinkedIn, floods with gleeful posts from white users showcasing their perfect AI action figures ("OMG look at me as an action figure!"), there's a darker side to this trend that nobody's talking about. ChatGPT 4o's image generation capabilities aren't the good for everyone at scale it's marketed to be.
The pattern is painfully obvious:
- White user uploads photo → Gets back close to perfect action figure replica
- Person of color uploads photo → Gets back......whatever stereotypical approximation the AI could muster.
My Personal AI Horror Story
When I prompted ChatGPT to "Take the original photo and make a photorealistic action figurine with my face on it. It could be called 'Cloud Jedi' and I have a light saber," the same prompt used by a white male LinkedIn user who posted his output with tons of comments high fiving the output, I wasn't expecting perfection. But damn! I certainly wasn't expecting to be aged decades and transformed into what looks like NVIDIA's CEO wearing a really bad black wig. I tested this prompt 3 times and each version of my action figure continued to degrade – getting older, shorter and stumpier. The only thing it got correct was the dress. Everything else – WRONG. Case in point, in the original picture, I’m not wearing glasses but in the AI version, my action hero is wearing glasses.
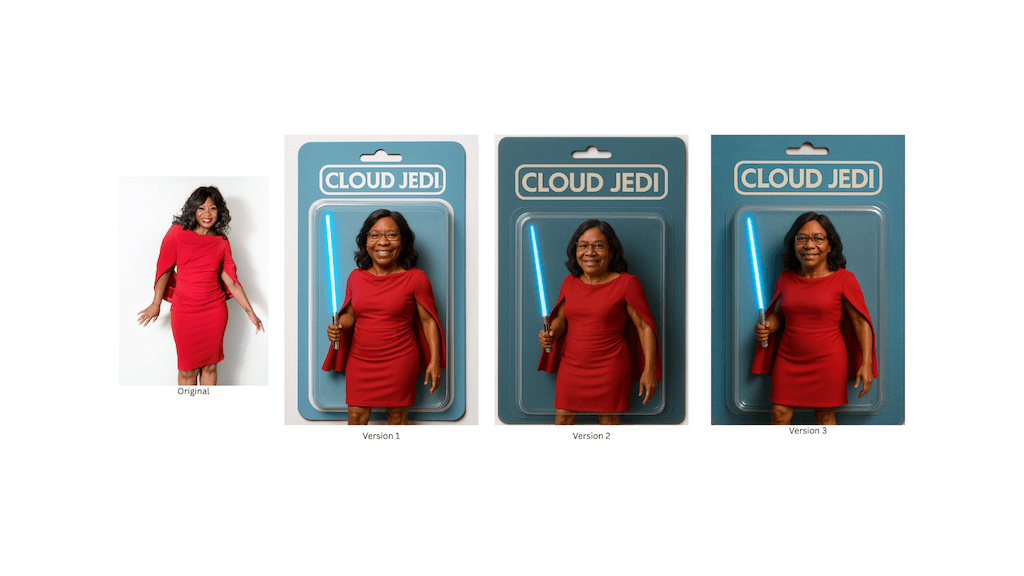
This isn't just "oh the AI made a mistake" territory. This is deeply concerning evidence of fundamental flaws in the training data. The system clearly hasn't been exposed to enough diverse faces to recognize and accurately represent people who don't fit the white default. It appears that the model trained on images, probably from those scraped off a lot of news sites. Other than Xi Jinping, what other Asian person has been in the news constantly for years? Jensen Huang. So, here we are.
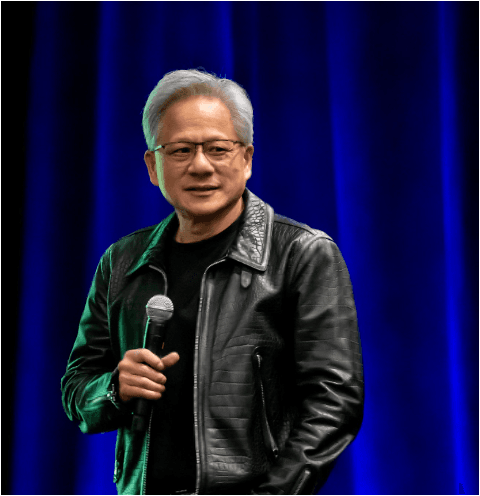
The Black Box Gets Blacker: OpenAI's Transparency Problem
And now, we learn that OpenAI has launched GPT-4.1 without the safety report that typically accompanies their model releases. According to OpenAI spokesperson Shaokyi Amdo, "GPT-4.1 is not a frontier model, so there won't be a separate system card released for it."
This attitude is particularly alarming when paired with what we're seeing in the action figure fiasco. How can we identify, address, and fix biases in systems that companies won't even document properly?
Former OpenAI safety researcher Steven Adler noted that system cards are "the AI industry's main tool for transparency and for describing what safety testing was done." Without them, we have no way of knowing what, if any, testing was done to ensure fair performance across demographics.
This comes at a time when OpenAI is already facing criticism from former employees about potentially cutting corners on safety work due to competitive pressures. The Financial Times recently reported that OpenAI has "slashed the amount of time and resources it allocates to safety testers."
The Compounding Crisis: Less Transparency + More Bias = Disaster
When you combine biased systems with decreased transparency, you create a perfect storm for technological discrimination:
- Hidden Biases Go Unfixed: Without transparency reports, developers can quietly ship biased systems with no public documentation of their limitations.
- No Accountability Mechanism: Companies can make vague promises about "improving" while continuing to deploy systems they know perform poorly for certain demographics.
- Safety Becomes Optional: As OpenAI has demonstrated by opposing California's SB 1047, which would have required safety evaluations, the industry is fighting against being held to consistent standards.
- Competitive Pressure Trumps Ethical Concerns: As companies race to release new models, safety and fairness testing becomes an expensive luxury rather than a requirement.
- Diminished Public Trust: When users like me see wildly inaccurate racial representations and then learn companies aren't even publishing safety information anymore, it destroys trust in the entire field.
The Urgent Need for Action
This isn't just about hurt feelings or disappointing toys. It's about fundamental representation in an AI-powered world where these systems increasingly determine how we're seen and understood. These failures reveal dangerous blind spots with far-reaching implications beyond novelty images.
Immediate Technical Interventions Needed
The AI industry must implement rigorous measures to address this systemic failure:
- Mandatory Safety Reporting:
- System cards must be required by law, not voluntary
- Reports must be published before model deployment, not weeks or months after
- Standard metrics must include performance across demographic groups
- Comprehensive Audit and Overhaul of Training Data:
- Independent third-party audits of all training datasets with transparent reporting of demographic representation
- Intentional collection of diverse facial datasets that properly represent global populations
- Documentation of representation metrics as a standard industry practice
- Diversity in Development Teams:
- Hiring practices that ensure AI teams reflect the diversity of potential users
- Inclusion of ethnically diverse testers in all phases of development
- Cultural competency training for all AI developers
- Testing Protocols That Center Marginalized Users:
- Implementation of "bias bounties" where users can report representational failures
- Pre-release testing focused specifically on historically marginalized groups
- Regular public transparency reports showing performance across demographic groups
- Meaningful Accountability Mechanisms:
- Financial consequences for companies that release demonstrably biased systems
- Regulatory frameworks that require equity in AI performance
- Consumer-friendly methods to report and track bias incidents
Beyond Technical Fixes
The problem runs deeper than code. We need cultural and structural changes:
- End the "Move Fast, Break People" Culture:
- The tech industry's "move fast and break things" mentality is particularly harmful when what's "breaking" is the dignity and representation of marginalized communities
- Companies must be held accountable when competitive pressure leads to cutting corners on safety and fairness testing
- Redefine "Good Performance":
- Success metrics must include equitable performance across all demographic groups
- No system should be considered "ready" if it works well only for majority populations
- End the practice of releasing systems with known demographic biases
- User Education and Empowerment:
- Clear communication about system limitations to all users
- Tools that allow users to identify and report bias
- Resources for understanding how bias manifests in AI systems
- Ethical Frameworks With Teeth:
- Industry-wide adoption of ethical AI principles that prioritize representational equity
- Concrete metrics to evaluate adherence to these principles
- External oversight bodies with enforcement capabilities
- Public Pressure When Voluntary Measures Fail:
- Support legislation like California's SB 1047 that would make safety evaluations mandatory
- Hold companies accountable when they fail to live up to their own stated principles
- Demand transparency as a prerequisite for public trust
The tech industry loves to talk about "moving fast and breaking things." But when the "things" being broken are the dignity and accurate representation of entire populations, we need to fundamentally reconsider our priorities.
An AI future where only certain people get to see themselves accurately reflected isn't innovation—its digital colonialism dressed up as progress.
The Real Question
While everyone's busy playing with their shiny new AI toys, we need to ask: who gets left out of the future we're building? And are we comfortable with a world where some people get accurate representations while others get crude approximations?
Next time you see those perfect ChatGPT action figures flooding your timeline, remember there's a whole segment of the population getting Jensen Huang'd instead. And maybe ask yourself: if the technology can't see us clearly, and the companies won't tell us how they're testing it, should anyone be using it at all?