AI in Finance: The Superpower We Didn’t Know We Needed—and the Cautionary Tales We Can’t Ignore
AI in Finance: Revolutionizing Financial Services Through Ethical Technology
The AI Financial Revolution
AI is a revolutionary force that is changing everything from fraud detection to client interaction in the finance industry today. Imagine a world where your bank account is as alert as a superhero from the Marvel Universe, spotting fraud before it takes place, personalizing services to suit your needs, and making banking as simple as making a coffee order. Nevertheless, tremendous power comes with great responsibility, and the financial stakes for AI are especially high.
AI has huge potential in the financial industry. It promises enhanced compliance, proactive fraud detection, and personalized support that transforms routine banking into meaningful encounters. But as much as AI holds the keys to a fairer system, there’s a flip side: poorly designed or biased algorithms can undermine the very trust they aim to build, creating financial harm and further entrenching systemic biases that hit vulnerable communities the hardest – at scale. This is the double-edged sword we must wield wisely.
AI's Role In Security: Enhancing Fraud Detection and Compliance
Banks have historically taken a reactive approach to fraud detection, identifying fraud after it has taken place. AI has transformed that playbook today. Advanced algorithms can detect anomalies before they become problematic since they can learn from millions of transactions in real time. When AI notices a pattern that would indicate fraud, such an odd withdrawal from a strange place, it alerts it right away. By increasing public trust and successfully protecting assets, this degree of proactive security turns financial stability from a "maybe" to a "given."
AI has changed what used to be an expensive and time-consuming process in compliance. AI transforms compliance from a reactive process to an always-on, active, and persistent safeguard by continuously monitoring transactions for regulatory adherence. This enhances accountability and transparency, which are the cornerstones for restoring trust in the financial services sector.
When AI Goes Wrong: The Apple Card Gender Bias Incident
However, AI has a darker side, which was made clear in 2019 by the Apple Card. Customers quickly noticed an unsettling pattern when Apple and Goldman Sachs debuted their card: even when their financial profiles were the same or better, women were frequently given lower credit limits than men. Despite their shared wealth, tech entrepreneur David Heinemeier Hansson stated that his wife's credit limit was 20x lower than his. As more women related similar situations, this revelation caused significant outrage.
Why? It seemed that the AI algorithms that set credit limits were biased against women. Whether intentional or not, this gender bias had a significant adverse financial impact, reducing women's financial options and perpetuating inequality on an enormous scale. The incident compelled Apple and Goldman Sachs to reassess their algorithms, illustrating the harsh reality of artificial intelligence: even a slight bias in the training data of a model can result in widespread discrimination. In this particular case, human prejudices were merely disguised by technology; it had not been removed.
This wasn’t the first, nor will it be the last, time an algorithm exposed inherent biases. AI models trained on historical data reflecting human biases—whether related to gender, race, or income—can inadvertently learn and replicate those biases. A model is just an abstract representation of reality, built on the data choices we make, reflecting our priorities and blind spots. Left unchecked, AI could codify financial discrimination into the very systems designed to democratize access.
The Issue of Exclusion: AI and the Unbanked
The potential for AI to unintentionally exclude people without access to traditional banking services is another significant concern. Due to socioeconomic constraints, inadequate infrastructure, and historical exclusionary practices, formal banking remains out of reach for millions of people worldwide, particularly in rural and low-income areas. These barriers disproportionately affect communities of color and women, who are often the most impacted by financial exclusion, with challenges compounded by cultural norms and gender inequality. Women, especially in developing regions, frequently rely on cash, informal lending circles, or community networks to meet their financial needs.
Since these individuals are "invisible" to the algorithms that drive today's financial systems, which assess risk, creditworthiness, and financial access utilizing indicators like bank activity, credit history, and official transactions, these individuals live in the shadow of datasets. Without these data sets, AI-powered models fail to include many women and underserved people, perpetuating a hard-to-break cycle of exclusion. Building the financial history required to become eligible for loans, credit, or even basic financial services is nearly impossible without access to traditional banking institutions.
The Role of Blockchain in Bridging the Gap
Blockchain offers a promising solution to this exclusion problem. With its decentralized nature, blockchain enables secure, transparent, and low-cost financial transactions without traditional banking intermediaries, making it especially useful in regions with limited infrastructure.
For example, Kenya’s blockchain-based platform BitPesa facilitates cross-border payments and remittances, offering faster and more affordable services than traditional methods. This has empowered many, especially women entrepreneurs, to engage in international trade and grow their businesses. During a recent visit to Kenya, I personally witnessed how the Masai tribe utilized these systems to support local economies and uplift communities.
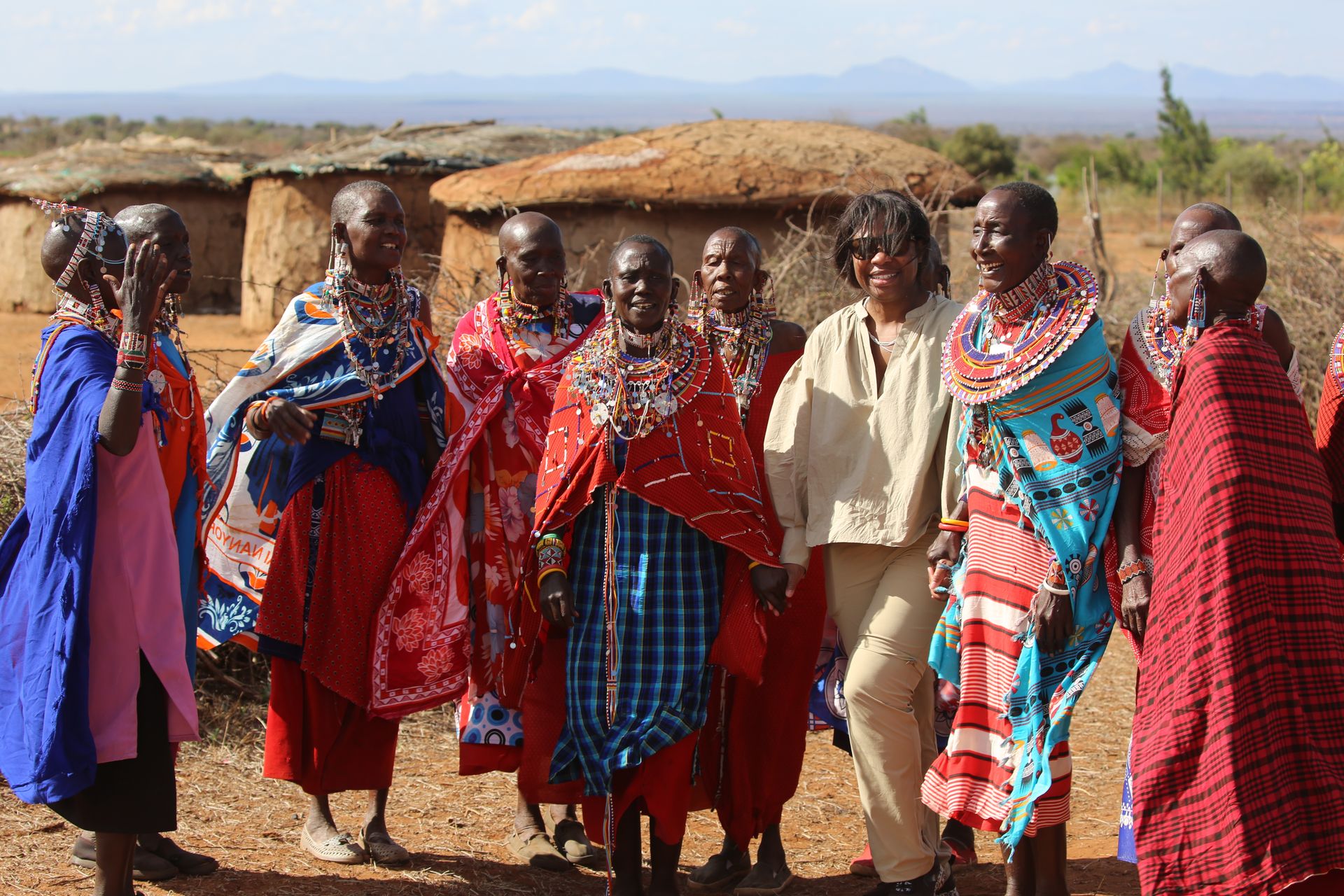
Beyond Blockchain: Innovative Solutions for Financial Inclusion
While blockchain is pivotal, other solutions are also closing the financial inclusion gap:
1. Mobile Money Services: Platforms like M-Pesa, launched in Kenya in 2007, have revolutionized financial access by enabling transactions via mobile phones. This innovation has provided essential services to unbanked populations, with a profound impact on women in rural areas.
2. Agent Banking: In India, the Business Correspondent (BC) model, or agent banking, employs local individuals to offer banking services in underserved areas. By March 2020, over 1.17 million BCs were delivering banking services across India, facilitating financial inclusion, especially during crises like COVID-19.
3. Digital Identity Solutions: Establishing a digital identity is crucial for accessing financial services. India’s Aadhaar system assigns unique IDs, allowing individuals to open bank accounts and access essential services. In April 2023 alone, Aadhaar facilitated over 1.96 billion transactions, demonstrating its widespread impact on financial inclusion.
4. Community-Based Savings Groups: In African and Asian countries, Village Savings and Loan Associations (VSLAs) allow members to pool resources and provide mutual loans. These self-managed groups have supported over 8 million members—primarily women—since 1991, offering critical financial services to those without formal banking.
Integrating AI with Alternative Data Sources
AI models must take into account alternative data which reflects the financial habits of individuals outside of traditional banking to be able to better serve unbanked populations. Financial institutions can offer products that are specifically designed for underserved communities and establish more inclusive credit scoring systems with the use of data such as utility payments, mobile phone usage, and community lending trends.
Biases Toward People of Color: A Systemic Challenge
AI holds the potential to eradicate discrimination in finance, but only if it is deployed judiciously. Inequalities are strengthened whenever algorithms trained on historical data inherit preexisting biases. Studies found that compared to their white peers with similar profiles, Black and Latino borrowers are more likely to encounter higher interest rates or loan denials. In one instance, a study conducted by the Federal Reserve Bank of Philadelphia found inherited biases were mostly to blame for racial differences in mortgage approvals.
Consider an AI system assessing loan risk. If the data suggests certain neighborhoods, often home to communities of color, are higher risk, AI will naturally skew its recommendations against these areas. According to Brookings, homes in Black neighborhoods are undervalued by 21% to 23% compared to similar homes in non-Black areas, costing these communities around $162 billion. This undervaluation leads to minority homeowners facing unfavorable loan terms or being denied refinancing options.
Moving Forward: Building Inclusive, Fair AI in Finance
To maximize AI’s strengths while minimizing risks, financial institutions need a multi-pronged approach:
1. Diverse Data: AI systems should be trained on representative datasets that reflect real-world diversity. Alternative data sources, such as rental history and utility payments, help provide a fuller picture for those lacking traditional credit history.
2. Bias Audits and Transparency: Regularly auditing for biases is essential. Financial institutions must also ensure transparency, allowing regulators and the public to understand AI decision-making processes.
3. Inclusive Design Teams: Diverse design teams can identify potential pitfalls and assess how models might impact different groups. This isn’t just ethical; it’s practical for creating accurate AI systems.
4. Community Partnerships: Collaborating with nonprofits and community groups can introduce financial services to unbanked communities in accessible ways, while also helping institutions understand these communities’ unique needs.
Building Financial Systems for All
The promise of AI in finance isn’t just about profit; it’s about creating a fairer, more inclusive system that serves everyone. AI’s power, when harnessed for good, can democratize access, but this requires more than good intentions. It requires transparency, vigilance, and a commitment to confronting our data’s hard truths.
A diversified strategy that makes use of cutting-edge models and technologies is required for financial inclusion. Digital identification solutions, agent banking, blockchain, mobile money, and community-based savings are all helping to close the gaps in financial inclusion. We can create a financial environment that genuinely benefits everyone, irrespective of their ability to use conventional banking services, by combining these technologies and creating AI systems with inclusivity in mind.
As financial institutions increasingly rely on AI, it’s essential to ask hard questions and take bold steps to ensure these tools empower rather than exclude. For example, do you have the right data, or are you substituting stand-in data or proxies to draw statistical correlations? Let’s build a future where financial security, access, and opportunity aren’t privileges but rights. Because when we harness AI for good, we’re not just changing an industry; we’re building a world where we rise the tide so everyone has a seat at the financial table.
©2025 Yvette Schmitter, All Rights Reserved